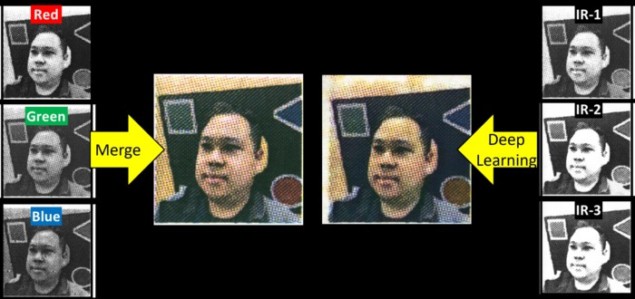
Infrared night-vision systems that see in colour could be a reality thanks to researchers in the US, who have used machine learning to create colour images of photographs that are illuminated with just infrared light. The team hope their technique could be further developed to create imaging systems that operate where the use of visible light is impossible, such as retinal surgery.
Traditional night vision systems work by illuminating an area with near infrared radiation and detecting the reflections or by using ultrasensitive cameras to detect the small amount of light present even at night. Both, however, usually produce monochromatic images, so researchers are seeking ways to produce multi-colour images of objects without having to bathe them in visible light.
Computer scientist Pierre Baldi of University of California, Irvine (UCI), explains that this would be very useful in medical applications where use of visible light is problematic. “It is very toxic for the retina to receive light of high intensity for long periods of time,” he explains. “Surgery may last four hours – if you have high intensity, short wavelength light shining on the retina continuously for four hours, there is a strong chance the patient may become blind. At the same time, you would like the surgeon to see things as though they were irradiated with regular light.”
Multi-wavelength infrared illumination
Researchers had previously tried several approaches to colourizing infrared images, but these often required some knowledge of the visible image as an input or sacrificed image clarity for colour. In this latest research, Baldi and ophthalmologist Andrew Browne, also at UCI, set out to produce full-colour images from multi-wavelength infrared illumination. This would allow images visually indistinguishable from the originals while obviating the use of visible light.
To achieve this, they first printed a rainbow colour palette from a standard, three-colour office printer onto white paper and imaged the spectral reflectance under multiple wavelengths of visible and near-infrared light. After selecting six wavelengths – three in the visible and three in the infrared – they printed 200 images of human faces from a public database and illuminated all of them with each wavelength in turn, detecting the intensity of the reflected light.
The researchers then developed a computational deep-learning algorithm, in which a computer predicted the reflectance at the three visible wavelengths using the values of the reflectance from the three infrared wavelengths. This generated an image that was then fed to a “discriminator” that evaluated the predicted image against the reference image and tried to tell them apart. If it could do so, this information was fed back to the generator, which used this information to refine its predictions.
“At the beginning of learning, when the parameters are sort of random, it produced a completely random type of image,” says Baldi. “There’s a procedure for adjusting the parameters of both the discriminator and the generator using this information.”
Ever-more accurate
The system was thereby trained to produce ever-more accurate colour reconstructions of the visible image from infrared data in a feedback loop. “The basic algorithms are the same as are being used in all computer vision tasks – whether it’s self-driving cars, looking at images from satellites or whatever, the same techniques can be used in all these imaging problems,” Baldi says.
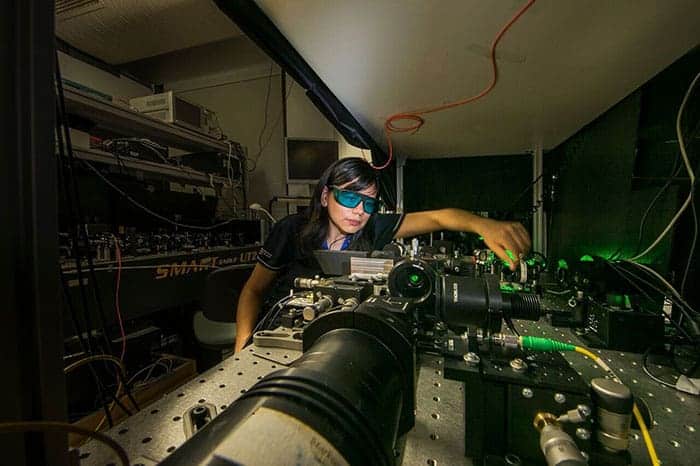
Semiconductor discs could boost night vision
At the end of the experiment, the researchers asked humans to evaluate the visual quality of the images produced by their deep learning algorithm. They found that the images were consistently rated as clearer and more accurate than those produced by a simple linear regression algorithm, in which the intensity at each visual wavelength in turn was predicted from the intensity at one infrared wavelength.
Two important improvements are needed before the system can be used for medical applications. One is to boost the current data acquisition rate beyond the current three frames-per-second so that video imaging is possible. The other is to adapt the system so that it works with biologically relevant samples such as retinal tissues – which have different infrared reflectance spectra than the images used in this study. Beyond this, the researchers suggest that the work could have applications in security and military operations and animal observation. “You can imagine many follow-up steps,” says Baldi.
The system is described in PLOS One.