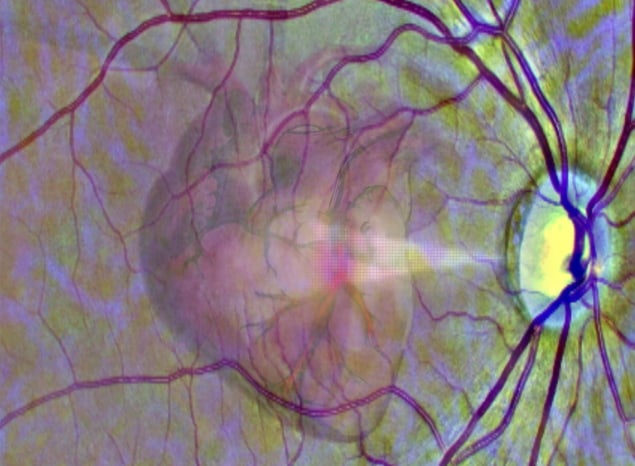
Retinal imaging is routinely performed by opticians to diagnose and monitor eye diseases and disorders. But retinal scans can reveal a lot more: retinal microvascular abnormalities may be indicative of broader vascular disease, including problems with the heart. An international, interdisciplinary research team headed up at the University of Leeds has now developed an artificial intelligence (AI) system that can automatically analyse these routine eye scans and identify individuals at risk of a future heart attack.
Cardiovascular disease is the leading cause of early death worldwide. Early identification and preventative treatment could help reduce its prevalence. Currently, a patient’s risk of cardiovascular disease is estimated using parameters including age, gender, smoking status and family history, along with medical imaging tests such as coronary CT, echocardiography and cardiovascular MRI. Such imaging procedures, however, are usually hospital-based and can be expensive, limiting their availability in countries with less well-resourced healthcare systems.
“This [AI-based] technique opens-up the possibility of revolutionizing the screening of cardiac disease,” explains senior author Alex Frangi in a press statement. “Retinal scans are comparatively cheap and routinely used in many optician practices. As a result of automated screening, patients who are at high risk of becoming ill could be referred to specialist cardiac services. The scans could also be used to track the early signs of heart disease.”
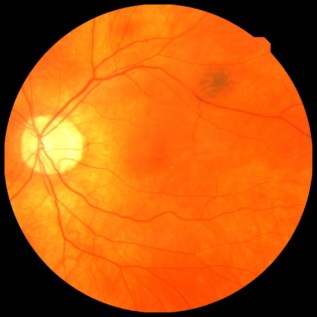
First author Andres Diaz-Pinto and colleagues investigated whether their AI system could use retinal images to estimate the mass and pumping efficiency of the heart’s left ventricle. An enlarged ventricle is linked with an increased risk of heart disease and can be used to predict the likelihood of a future heart attack, or myocardial infarction (MI).
To achieve this, the researchers trained a multichannel variational autoencoder and a deep regression network to estimate the left ventricular end-diastolic volume (LVEDV) and left ventricular mass (LVM) directly from retinal images. They trained and validated the two networks in this AI system on data from 5663 and 71,515 participants, respectively, from the UK Biobank Imaging Study.
The first data set had cardiac MR images, high-quality retinal images and demographic data, while the latter had only high-quality retinal scans and demographic data. Comparison with ground-truth delineations of the cardiovascular MR images demonstrated that the retinal images could be used to quantify these cardiac parameters.
Next, the researchers used the estimated LVM/LVEDV combined with the demographic data, as well as the demographics alone, to predict whether a patient was at risk of a heart attack over the subsequent 12 months. For this comparison, they used data from UK biobank participants with retinal images that weren’t used for the AI training: 992 cases in which MI had occurred after the images were taken and 992 non-MI cases.
They found that adding the estimated LVM/LVEDV to the demographic data improved the AI system’s predictive performance. The system predicted future MI events from retinal images with a sensitivity and specificity of 0.74 and 0.72, respectively, with just age and gender as additional variables (as would be available in an opticians or eye clinic).
Finally, the team performed an external validation using retinal images and demographic data from an independent dataset – the NIH Age-Related Eye Disease Studies – containing 180 participants with MI events and 2830 without. They found that the algorithm’s ability to predict MI was impacted by the presence of age-related macular degeneration (AMD) in the retinal images.
The predictive performance of the AI system was highest in cases without AMD, where the sensitivity and specificity were 0.70 and 0.67, respectively, and decreased in individuals with AMD of increasing severity. The team notes that the presence of retinal disease such as AMD, particularly in its more severe forms, interferes with the algorithm’s ability to infer systemic circulation characteristics from the retinal circulation.

Eye scan can detect signs of Alzheimer’s disease
The researchers conclude that AI system can estimate cardiac parameters and predict future MI events using inexpensive and easy-to-obtain retinal photographs and demographic data. They suggest that their approach could be used in eye clinics and opticians to identify patients at risk of future MI events and refer them for in-depth cardiovascular investigation.
The study is described in Nature Machine Intelligence.