Elekta is betting on big-data technologies and cloud-based software innovation to rewrite the rules of precision radiation medicine

The convergence of big-data analytics with secure and scalable cloud-based data storage presents an inflection point in radiation oncology – an opportunity to reimagine operational best practice in the planning, delivery and management of radiation therapy while personalizing cancer treatment to meet the clinical needs of individual patients. It’s a compelling vision, one that sits front-and-centre within Elekta’s technology roadmap and – more specifically – informs the development of ProKnow, a suite of cloud-based software tools that enables radiation oncology clinics – and, more broadly, regional and international oncology networks – to aggregate, structure and interrogate their diverse, and previously fragmented, data stores.
Think consolidated data warehousing – at terabyte scale and beyond. Think geographically distributed access to that data warehouse via a simple web-based user interface. In this way, ProKnow allows clinical teams to retrieve and analyse patient DICOM data along a range of coordinates – imaging, planning, treatment modality and delivery system – and share those data sets with colleagues across departments and in other treatment centres. Think remote, collaborative, always-on data access. “Those collective data streams create a cloud-based knowledge repository that oncology teams can mine for clinical insights – trends, relationships, outliers, significance – to support innovation and continuous improvement in patient care,” says Ben Nelms, founder of ProKnow and adviser to Elekta on the ProKnow development programme.
Underpinning it all, given the cloud-based architecture of ProKnow, is bulletproof cybersecurity, with all patient data encrypted in transit and at rest in a fully HIPAA-compliant environment (ensuring the privacy and integrity of sensitive patient information). In terms of user access, for example, ProKnow supports multifactor authentication, as well as single sign-on for institutions that have established their own federated user authentication. “ProKnow is as secure as online banking – if not more secure,” claims Nelms, “so that accredited clinical users can log on and authenticate from anywhere as long as they have a WiFi connection.”
Clinical innovation
Operationally, it’s clear that collaboration and peer review are hard-wired into the ProKnow value proposition. After all, this is a software platform designed to harness the collective domain knowledge and expertise scattered across multiple clinical departments and multiple treatment centres. That means clinicians, physicists, dosimetrists and other experts are able to evaluate critical anatomy contouring, treatment design and plan quality as team activities – sharing the load and distributing tasks as needed.
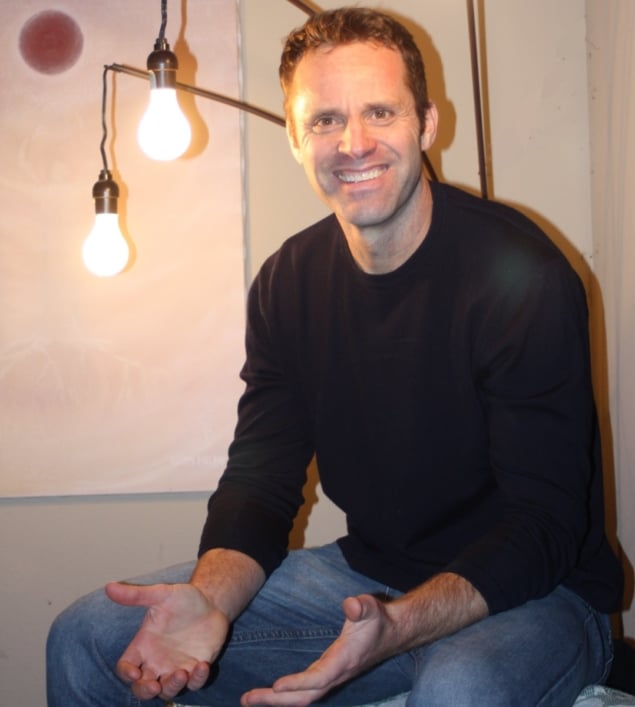
“By centralizing data and opening up access, specialists are now accessible across geographically distributed ProKnow clinics,” explains Nelms. “In short, there’s no reason why clinical experts in, say, hypofractionated radiotherapy for lung cancer or MR-Linac treatment of pelvic malignancies should be constrained to a single hospital.”
In the short term, ProKnow can help the radiation oncology team create a unified framework for treatment planning, driving standardization and best practice across collaborating treatment facilities. It is possible to take a single patient plan – for example, a stereotactic radiosurgery (SRS) treatment for a complex cranial indication – and benchmark against a repository of previous similar cases using a range of predefined metrics such as dose coverage, tissue sparing, dose-volume histogram (DVH) and planning times. “Users can customize these critical metrics and their clinical objectives into scorecards, then extract for individual patients as well as across populations,” says Nelms. “From here, clinicians are able to rate the current patient’s plan quality and address any unwanted variations.”
Subsequently, those scorecards can be shared across treatment centres to give planners a standardized baseline from which to develop individualized treatment plans on a patient-by-patient basis. The emphasis on standardization extends to atlases of best-practice organ contouring, region-of-interest nomenclature and peer-review task management. During routine plan review, for example, a clinician might be concerned by a seemingly high mean dose to the brain stem. “With ProKnow,” says Nelms, “it’s a straightforward exercise to review similar patient treatment plans over a defined timeframe, calling up relevant histograms and scatter plots to allow comparison and iteration.”
It’s all about outcomes
Another innovative feature of ProKnow is the ease with which patients can be assigned into one or more cohorts. These user-defined populations of similar patients enable clinicians to track treatment efficacy and outcomes over time. In other words, ProKnow is shaping up as a core platform technology for individual clinics – as well as multicentre cancer networks – to conduct their own clinical trials using data they’re generating as part of the day-to-day treatment workflow.
“When users centralize data, they’re effectively building up a powerful asset that they can learn from,” says Nelms. “It’s all about mining the data to figure out what’s working best in terms of patient outcomes and workflow efficiency.” A case in point is the clinical roll-out and validation of machine-learning technologies for autosegmentation and automated treatment planning, with ProKnow facilitating “analysis-of-variance” comparisons with prior patient cohorts based on manual contouring and planning.
It’s similarly straightforward to integrate custom metrics not found in the patient DICOM data – including measures of success or toxicity as well as variables such as drug regimens, genetic factors, immobilization techniques and image-guidance technologies. “With this multichannel data aggregation,” says Nelms, “users can continually look for statistical relationships between patient outcomes and DVH and dose-based metrics as well as other predictive factors.”
- For further information, view the Physics World webinar ProKnow: distribute tasks, centralize knowledge and harness the power of big data.